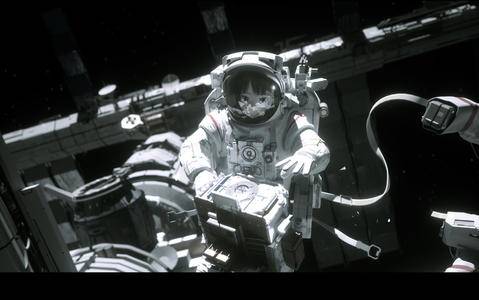
🔥人工智能
笔记和资料,涉及到深度学习、自动驾驶等领域。
🔥机器人
包括ROS机器人框架笔记。Beginer Friendly
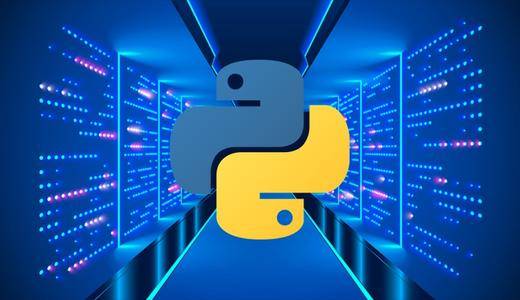
✅Python教程
从0到1,在深入人工智能的全套Python笔记。
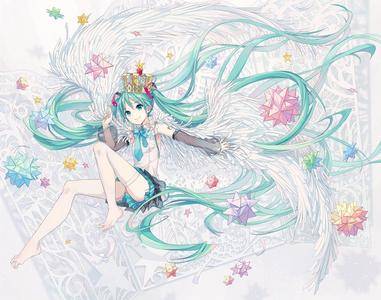
❤️经验经历
过往的感悟和思考。发病日记。
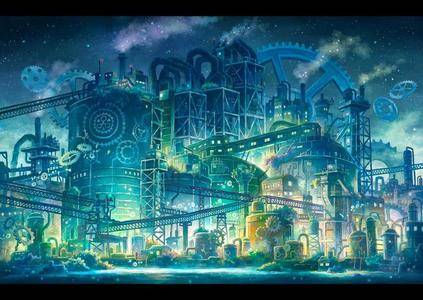
✨碎片技术
学习工作中遇到的很赞的技术碎片,整理好了。
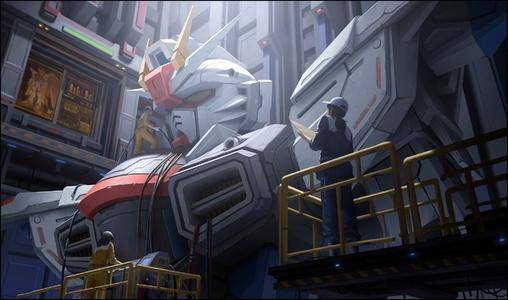
✨学习积累
相对于碎片技术的,已经沉淀为自己的资本的内容。
SP Module 6 Speech Synthesis – Waveform Generation and Connected Speech
DiphonePhones are not a suitable unit for waveform concatenation, so we used diphones, which capture co-articulation.
Diphone starts at the middle of one phone and ends at the middle of the other.
Coarticulation is the overlapping of adjacent articulations or the influence of the target phoneme on surrounding phonemes. Middles of phones are more stable in their spectral properties than the edges, because of coarticulation. So, concatenating diphones should lead to smoother joins
Waveform conca ...
SP Module 5 Speech Synthesis – Phonemes and the Front End
Tokenisation & normalisation标签化 & 正则化
When processing almost any text, we need to find the words. This involves splitting the input character sequence into tokens and normalising each token into words.
Handwritten rulesEvery user of a language holds a lot of knowledge about that language in their mind. One way to capture and make use of that knowledge is in the form of rules.
Finite state transducerFinite State Transducers provide general-purpose machinery for rewriting an input sequen ...
MOB LEC8 Recursive and Kalman Filter
Prerequisite knowledgestates of mobile robot, motion model, position orientation and velocity,
More challenge see: GPS
Kalman FilterPredict, measure, combining
Prediction and correction
Linear Kalman Filter
Recursive Least Squares + Process Model
Extended Kalman FilterLinear approximation, first-order term, still linear.
Linearized motion model, Linearized measurement model.
Jacobian matrix
Limitation of Kalman Filter
Summary
The Kalman Filter is very similar to RLS but includes a motion mode ...
MOB LEC7 Semantic Segmentation
Problem Formulationpixel-level prediction
Challenge of Semantic Segmentation
General vision challenges: occlusion, truncation, scale and illumination changes
Challenges specific to segmentation: smooth boundaries are hard to obtain due to the intrinsic ambiguity and resolution limitation in the image space.
Segmentation with DNN
Semantic Segmentation for Scene Understanding
RANSAC algorithm
boundaries
Additional materialTrue Positive, False Positive, False Negative
individual object
help ...
SP Modules Review Contents
Module 1: Phonetics and Representations of SpeechSystems in Speech ProductionSpeech production involves three systems in the body: the respiratory system, the phonation system, and the articulation system (Figure 1.2).
The Respiratory SystemThe respiratory system supplies the air needed to initiate speech sounds (see Figure 1.3). It consists of parts of the body that allow us to breathe, including the lungs, the diaphragm, the muscles of the rib cage, and the abdominal muscles.
The Phonation S ...
SP Module 4 the Source-Filter Model
HarmonicsIn the frequency domain, periodic signals have harmonic structure: they contain energy only at multiples of their fundamental frequency.
Voice sounds different from unvoiced sounds, has repeating pattern, in periodicity. So, the peak of the sound in the frequency domain is clear to observe.
Impulse trainAn impulse train is the simplest periodic signal that has energy at all multiples of its fundamental frequency (and energy are evenly distributed).
Spectral envelopeVarying the shape o ...
PI Week6 the Human Element
Introduction
This week we continue the theme of looking at general kinds of harm technology can cause if we’re not careful with it.
The topic we’re looking at is one that, with a few exceptions, gets a lot less press I think than bias because it’s a lot harder to quantify (and as established in week 1, computer scientists love quantitative data)
That topic is human beings, and specifically what we might unintentionally affect when we remove people from a system, or at least change their involvem ...
PI Week5 Bias and Fairness
Introduction
We’ve covered some important background concepts in talking about Responsibility, Power and Data. The idea behind these has been to motivate some of why we should be interested in ethics in computer science, and why we are often going to be at least partially responsible for anticipating and mitigating harms that technical artifacts can bring about.
For this middle portion of the course, we are looking at some broad categories of harm (some of the Data readings begun this process). ...
PI Week4 Data Ownership
The increasing availability of digital data reflecting economic and human development, and in particular the availability of data emitted as a by-product of people’s use of technological devices and services, has both political and practical implications for the way people are seen and treated by the state and by the private sector. Yet the data revolution is so far primarily a technical one: the power of data to sort, categorise and intervene has not yet been explicitly connected to a social ju ...
PI Week3 Power
Definition
Your ability to see your will made manifest in the world.
Explanation
If you want to do something, how easy is it for you to do it. To naively put this in informatics terms, you could think of it as the weight associated with a person’s node in a network.
Because we live in a world of systems (political, legal, cultural, organisational, social), a lot of that is tied up in how those system are designed. And a lot of that is dependent on who they were designed for, or by. Because, int ...
MOB LEC6 Object Detection
Challenge of object detectionnot fully observed, scale distraction, illumination changes.
Basic conceptsbounding box and class labels,
intersection of union (IoU)
See more at: Evaluationg Mateics
2D Object Detection Steps (inference)feature extractor, computationally expensive, lower widthe and height, greater depth
Prior bounding boxes, or anchor bounding boxes, assume bounding boxes, then guess where and how large they are.
centroid location (where), box dimensions (size)
Every pixel in ...
MOB LEC5 Feed Forward Neural Network
Since most material has been covered in previous blogs, I will go through this lecture contents in brief.
Brief overview
More ContentsActivation function example
Task example
For classification we have:
Softmax output layer
Cross-entropy Loss function
For regression we have:
Linear output layer
Mean square error lLoss function
Supplement reading
LeCun, Yann, Yoshua Bengio, and Geoffrey Hinton. “Deep learning.” nature 521.7553 (2015):436-444.
A Comprehensive Guide to Convolut ...
MOB LEC4 Image Feature Matching
Image Features: A General Process
Step 1 - Feature Detection: identify distinctive points in our images. We call these points features.
Step 2 - Feature Description: associate a descriptor for each feature from its neighborhood.
Step 3 - Feature Matching: we use these descriptors to match features across two or more images.
Feature DetectionFeature Define
Features: Points of interest in an image defined by its image pixel coordinates [u, v].
Points of interest should have the following ch ...
MOB LEC3 Cameras and Images
Introduction of computer visionComputer vision is a field of artificial intelligence (AI) that enables computers derive meaningful information from digital images, videos and other visual inputs obtained by a camera.
Bucket of photons
Photons converted to electrons
Shift electrons along row for readout
The readout on the device will translate the analog signal to either grayscale images or RGB images
Image Formation
pinhole camera, optical centre, focal length
Stereo Cameras
Image filter ...
SP Module 3 – Digital Speech Signals
Time domainSound is a wave of pressure travelling through a medium, such as air. We can plot the variation in pressure (captured by microphone) against time to visualise the waveform.
Sound sourceAir flow from the lungs is the power source for generating a basic source of sound either using the vocal folds or at a constriction made anywhere in the vocal tract.
somehthing about pressure with our vocal folds, the air flow is slow, its only the power source of sound, the pressure change is the ke ...